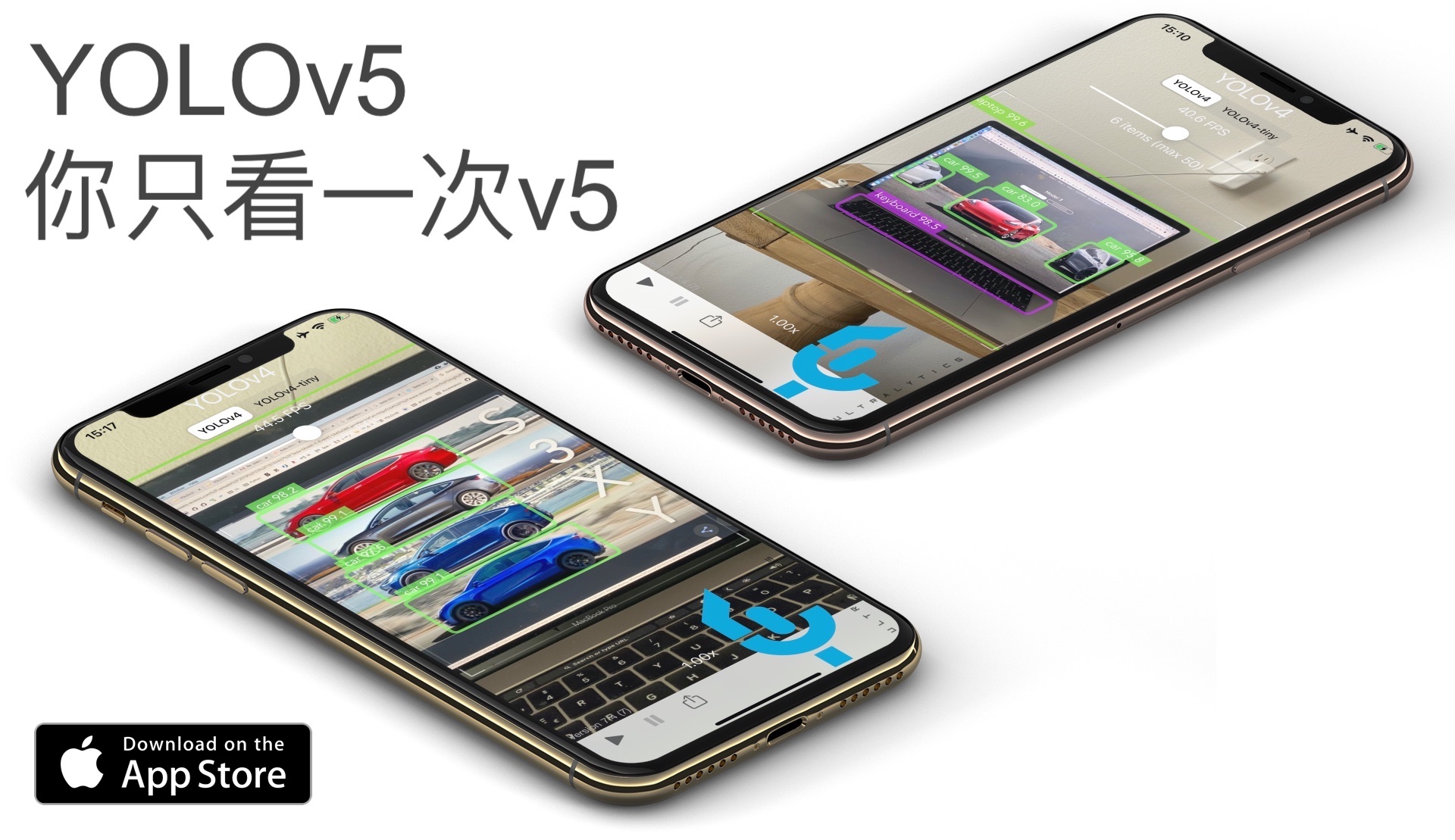
This repo only focuses on NMS speed improvement based on https://github.com/ultralytics/yolov5.
See non_max_suppression
function of utils/general.py for our Cluster-NMS implementation.
Torchvision NMS has the fastest speed but fails to run in batch mode.
Batch mode Cluster-NMS is made for this.
Our goal is that when using TTA for getting better performance, NMS no longer becomes a potential time-consuming growth factor.
Model | APval | APtest | AP50 | SpeedGPU | FPSGPU | params | FLOPS | |
---|---|---|---|---|---|---|---|---|
YOLOv5s | 37.0 | 37.0 | 56.2 | 2.4ms | 416 | 7.5M | 13.2B | |
YOLOv5m | 44.3 | 44.3 | 63.2 | 3.4ms | 294 | 21.8M | 39.4B | |
YOLOv5l | 47.7 | 47.7 | 66.5 | 4.4ms | 227 | 47.8M | 88.1B | |
YOLOv5x | 49.2 | 49.2 | 67.7 | 6.9ms | 145 | 89.0M | 166.4B | |
YOLOv5x + TTA | 50.8 | 50.8 | 68.9 | 25.5ms | 39 | 89.0M | 354.3B | |
YOLOv3-SPP | 45.6 | 45.5 | 65.2 | 4.5ms | 222 | 63.0M | 118.0B |
For more details, please refer to https://github.com/ultralytics/yolov5.
Python 3.8 or later with all requirements.txt dependencies installed, including torch>=1.6
. To install run:
$ pip install -r requirements.txt
- 1 RTX 2080 Ti
Evaluation command: python test.py --weights yolov5s.pt --data coco.yaml --img 640 --augment --merge --batch-size 32
YOLOv5s.pt
NMS | TTA | max-box | weighted threshold | time (ms) | AP | AP50 | AP75 | APs | APm | APl |
---|---|---|---|---|---|---|---|---|---|---|
Torchvision NMS | on | - | - | 3.2 / 17.9 | 38.0 | 56.5 | 41.2 | 20.9 | 42.6 | 51.7 |
Merge + Torchvision NMS | on | - | 0.65 | 3.2 / 18.6 | 38.0 | 56.5 | 41.4 | 20.9 | 42.7 | 51.8 |
Merge + Torchvision NMS | on | - | 0.8 | 3.2 / 18.9 | 38.1 | 56.5 | 41.4 | 21.0 | 42.7 | 51.8 |
Weighted Cluster-NMS | on | 1000 | 0.8 | 3.2 / 6.6 | 38.0 | 55.7 | 41.6 | 20.5 | 42.8 | 51.9 |
Weighted Cluster-NMS | on | 1500 | 0.65 | 3.2 / 10.2 | 38.1 | 56.1 | 41.9 | 20.9 | 42.7 | 51.8 |
Weighted Cluster-NMS | on | 1500 | 0.8 | 3.2 / 10.2 | 38.3 | 56.2 | 41.8 | 21.1 | 43.0 | 52.0 |
Weighted Cluster-NMS | on | 2000 | 0.8 | 3.2 / 14.5 | 38.4 | 56.4 | 41.9 | 21.3 | 43.1 | 52.1 |
Torchvision NMS | off | - | - | 1.5 / 5.4 | 36.9 | 56.2 | 40.0 | 21.0 | 42.1 | 47.4 |
Merge + Torchvision NMS | off | - | 0.65 | 1.3 / 6.7 | 36.9 | 56.2 | 40.2 | 20.9 | 42.1 | 47.4 |
Merge + Torchvision NMS | off | - | 0.8 | 1.3 / 6.7 | 37.1 | 56.2 | 40.3 | 21.1 | 42.2 | 47.6 |
Weighted Cluster-NMS | off | 1000 | 0.65 | 1.3 / 6.5 | 36.9 | 56.0 | 40.2 | 20.9 | 42.0 | 47.3 |
Weighted Cluster-NMS | off | 1000 | 0.8 | 1.3 / 6.5 | 37.0 | 56.0 | 40.3 | 21.1 | 42.2 | 47.5 |
YOLOv5m.pt
NMS | TTA | max-box | weighted threshold | time (ms) | AP | AP50 | AP75 | APs | APm | APl |
---|---|---|---|---|---|---|---|---|---|---|
Torchvision NMS | on | - | - | 6.4 / 10.4 | 45.1 | 63.2 | 49.0 | 27.0 | 50.2 | 60.5 |
Merge + Torchvision NMS | on | - | 0.65 | 6.4 / 11.5 | 45.0 | 63.2 | 49.0 | 26.9 | 50.2 | 60.3 |
Merge + Torchvision NMS | on | - | 0.8 | 6.4 / 11.5 | 45.2 | 63.3 | 49.1 | 27.0 | 50.3 | 60.5 |
Weighted Cluster-NMS | on | 1000 | 0.65 | 6.4 / 6.8 | 44.6 | 62.3 | 49.1 | 26.0 | 50.0 | 60.4 |
Weighted Cluster-NMS | on | 1500 | 0.65 | 6.4 / 9.8 | 44.9 | 62.9 | 49.4 | 26.6 | 50.2 | 60.4 |
Weighted Cluster-NMS | on | 1500 | 0.8 | 6.4 / 9.8 | 45.2 | 62.9 | 49.4 | 26.8 | 50.4 | 60.5 |
Torchvision NMS | off | - | - | 2.7 / 4.5 | 44.3 | 63.2 | 48.2 | 27.4 | 50.0 | 56.4 |
Merge + Torchvision NMS | off | - | 0.65 | 2.7 / 6.1 | 44.2 | 63.1 | 48.4 | 27.4 | 50.1 | 56.2 |
Merge + Torchvision NMS | off | - | 0.8 | 2.7 / 6.1 | 44.4 | 63.2 | 48.6 | 27.6 | 50.2 | 56.4 |
Weighted Cluster-NMS | off | 1000 | 0.65 | 2.7 / 6.1 | 44.2 | 62.9 | 48.5 | 27.3 | 50.0 | 56.3 |
Weighted Cluster-NMS | off | 1000 | 0.8 | 2.7 / 6.1 | 44.3 | 62.9 | 48.5 | 27.4 | 50.1 | 56.4 |
YOLOv5x.pt python test.py --weights yolov5s.pt --data coco.yaml --img 832 --augment --merge --batch-size 32
NMS | TTA | max-box | weighted threshold | time (ms) | AP | AP50 | AP75 | APs | APm | APl |
---|---|---|---|---|---|---|---|---|---|---|
Merge + Torchvision NMS | on | - | 0.65 | 31.7 / 10.7 | 50.2 | 68.5 | 55.2 | 34.2 | 54.9 | 64.0 |
Weighted Cluster-NMS | on | 1500 | 0.8 | 31.8 / 9.9 | 50.3 | 68.0 | 55.4 | 33.9 | 55.1 | 64.6 |
- AP reports on
coco 2017val
. TTA
denotes Test-Time Augmentation.max-box
denotes maximum number of boxes processed in Batch Mode Cluster-NMS.weighted threshold
denotes the threshold used in weighted coordinates.- time reports model inference / NMS.
- To avoid randomness, NMS runs three times here. See test.py.
# Run NMS
t = time_synchronized()
output = non_max_suppression(inf_out, conf_thres=conf_thres, iou_thres=iou_thres, max_box=max_box, merge=merge)
output = non_max_suppression(inf_out, conf_thres=conf_thres, iou_thres=iou_thres, max_box=max_box, merge=merge)
output = non_max_suppression(inf_out, conf_thres=conf_thres, iou_thres=iou_thres, max_box=max_box, merge=merge)
t1 += time_synchronized() - t
- Batch mode Weighted Cluster-NMS will have comparable speed with Torchvision merge NMS when batchsize>=16 and without TTA.
- When using TTA, the time of torchvision NMS will increase significantly, because the model predicts much more boxes. Especially when using multi-scale testing or more TTA means.
- Observed from experience, when using TTA, max-box = 1500 will be good. And when TTA is turned off, max-box = 1000.
YOLOv5 may be run in any of the following up-to-date verified environments (with all dependencies including CUDA/CUDNN, Python and PyTorch preinstalled):
- Google Colab Notebook with free GPU:
- Kaggle Notebook with free GPU: https://www.kaggle.com/ultralytics/yolov5
- Google Cloud Deep Learning VM. See GCP Quickstart Guide
- Docker Image https://hub.docker.com/r/ultralytics/yolov5. See Docker Quickstart Guide
- Distance-IoU Loss: Faster and Better Learning for Bounding Box Regression
- Enhancing Geometric Factors into Model Learning and Inference for Object Detection and Instance Segmentation
@Inproceedings{zheng2020diou,
author = {Zheng, Zhaohui and Wang, Ping and Liu, Wei and Li, Jinze and Ye, Rongguang and Ren, Dongwei},
title = {Distance-IoU Loss: Faster and Better Learning for Bounding Box Regression},
booktitle = {The AAAI Conference on Artificial Intelligence (AAAI)},
year = {2020},
}
@Article{zheng2021ciou,
author = {Zheng, Zhaohui and Wang, Ping and Ren, Dongwei and Liu, Wei and Ye, Rongguang and Hu, Qinghua and Zuo, Wangmeng},
title = {Enhancing Geometric Factors in Model Learning and Inference for Object Detection and Instance Segmentation},
booktitle = {IEEE Transactions on Cybernetics},
year = {2021},
}